Lightning Talk at Learning on Graphs Conference @ Cambridge Meetup
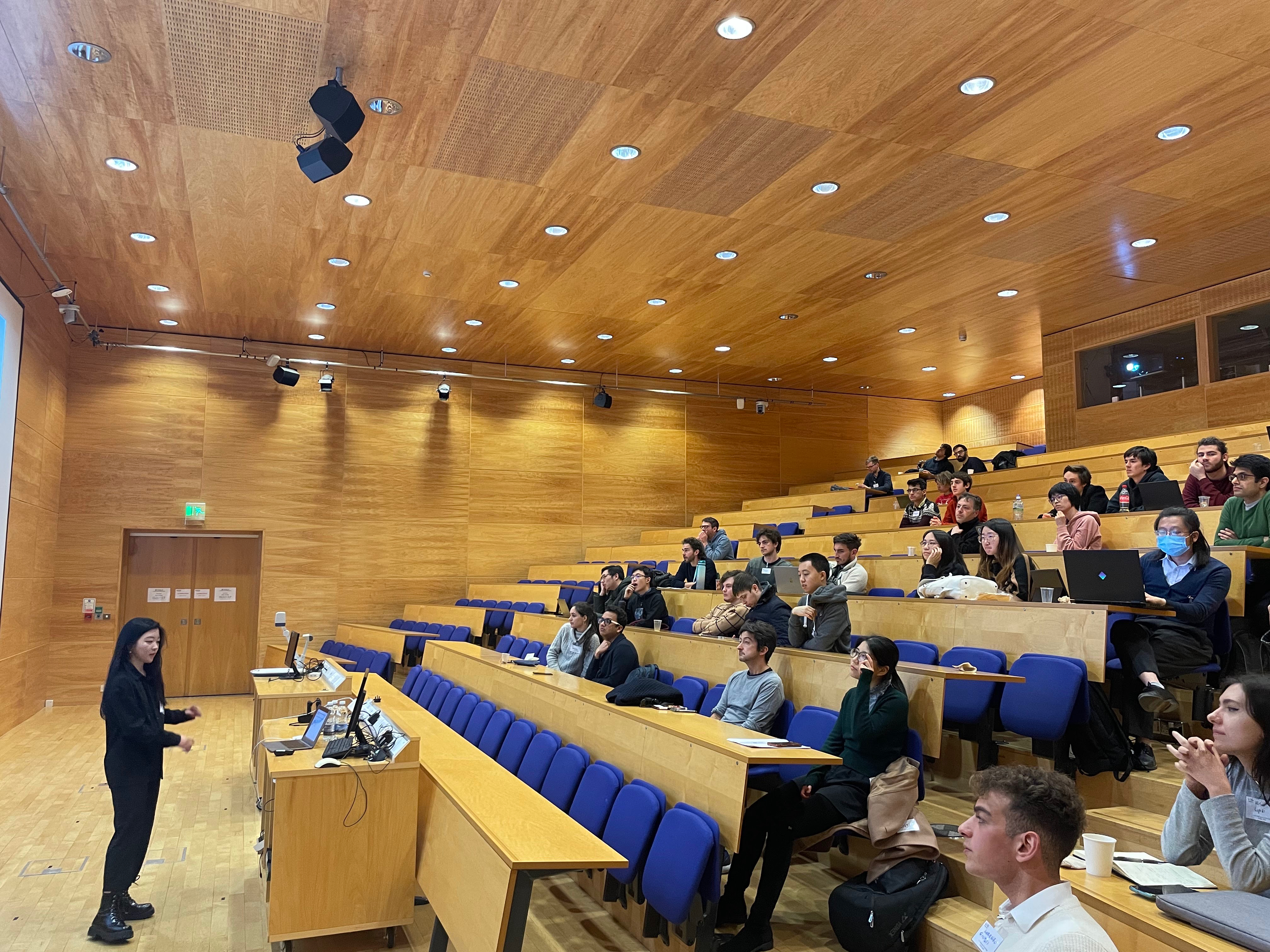
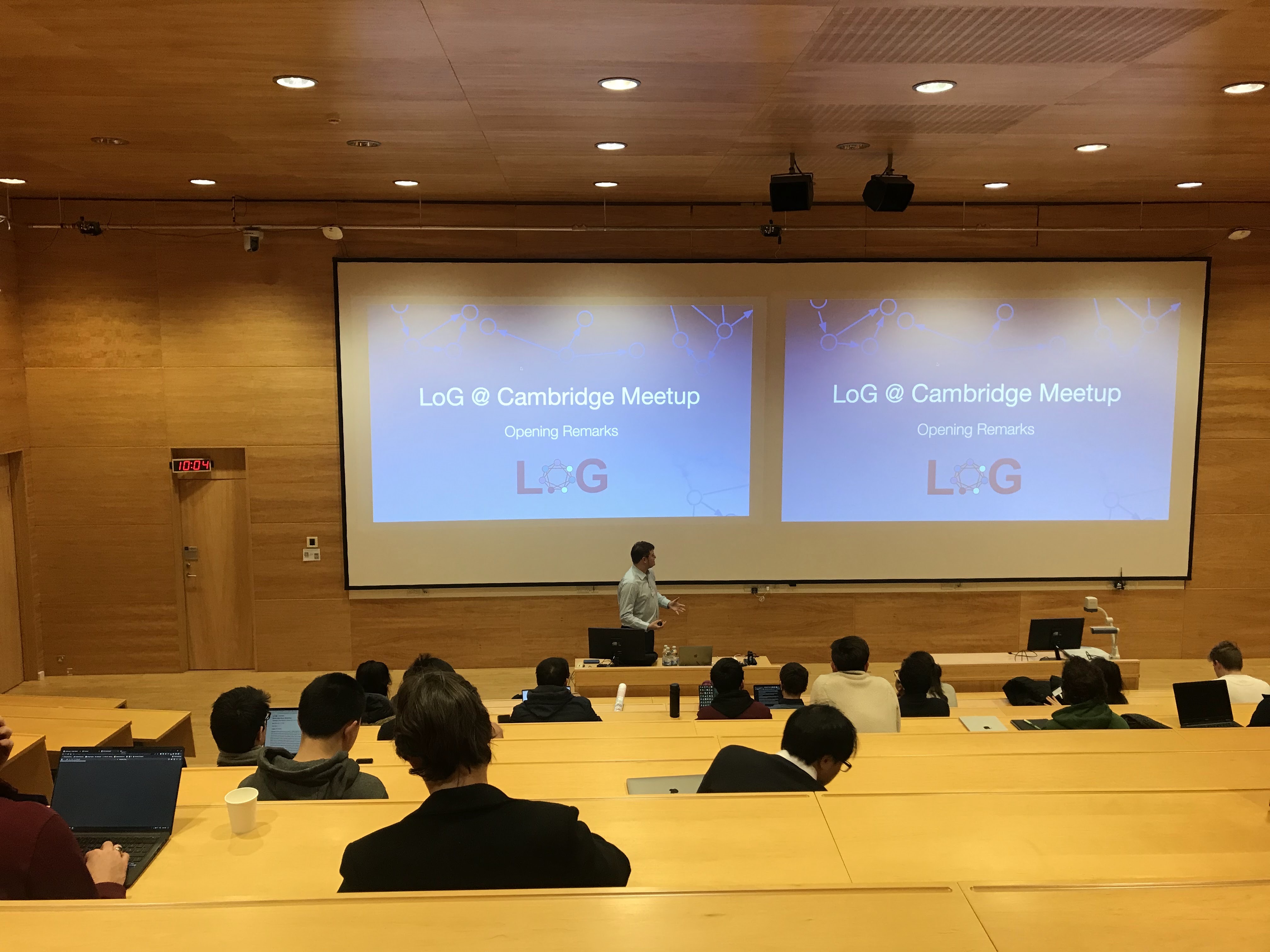
On December 10, 2022, I had the honor of speaking at the Learning on Graphs Conference Meeting in Cambridge organized by Professor Petar Veličković from DeepMind. I shared my paper with researchers from academia and industry.
Motivation of the project is a fusion of causality and graph neural networks designed to disentangle feature correlation to improve the interpretability of representation learning. The novelty comes in the creation of the graph, which uses the Bayesian Network's connectivity to establish the graph's edge and the Conditional Average Treatment Effect as the weight to attribute the graph's node in order to accomplish graph representation learning.
AI for Sustainable Development Goals Project Proposal Competition @ UNDP
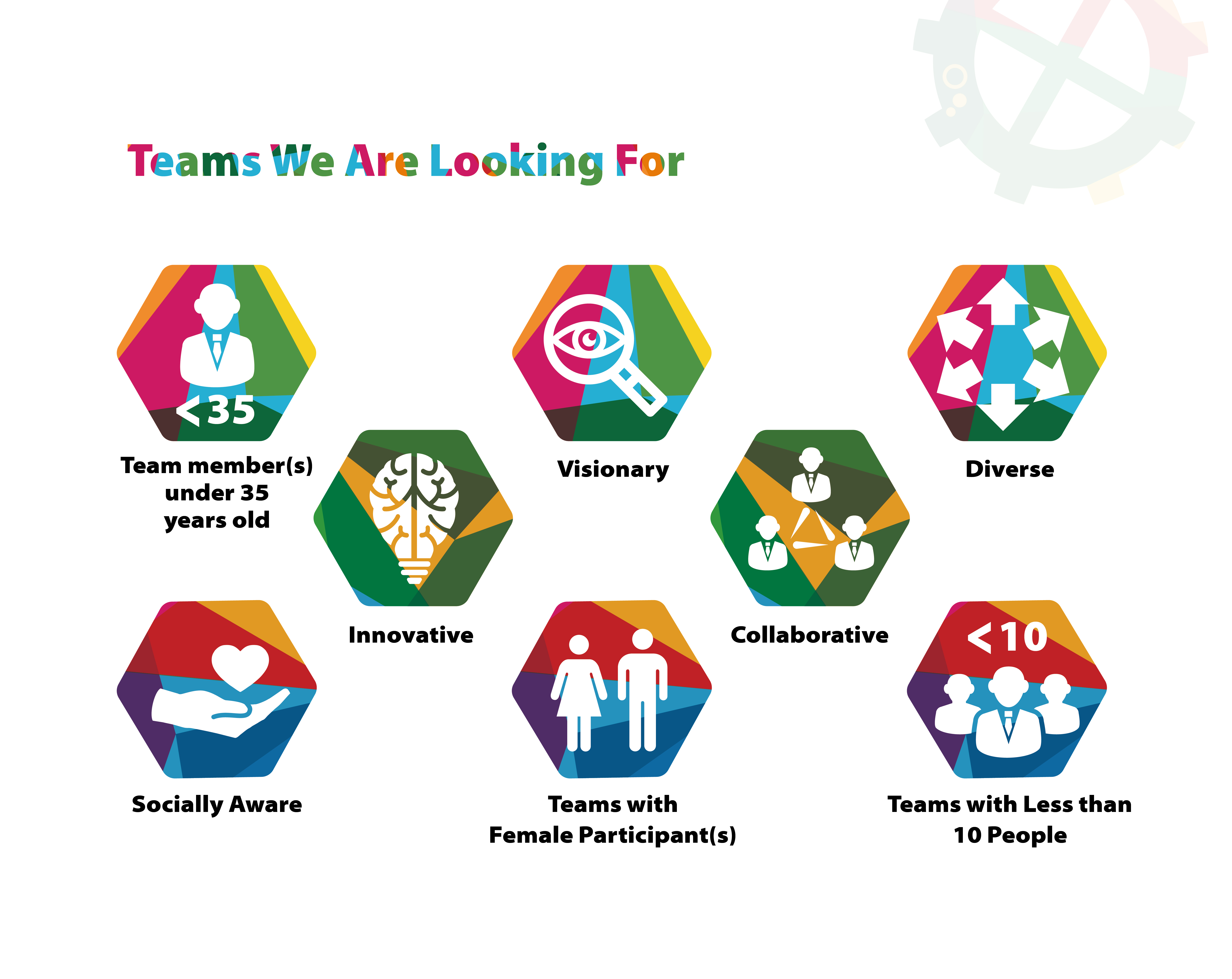
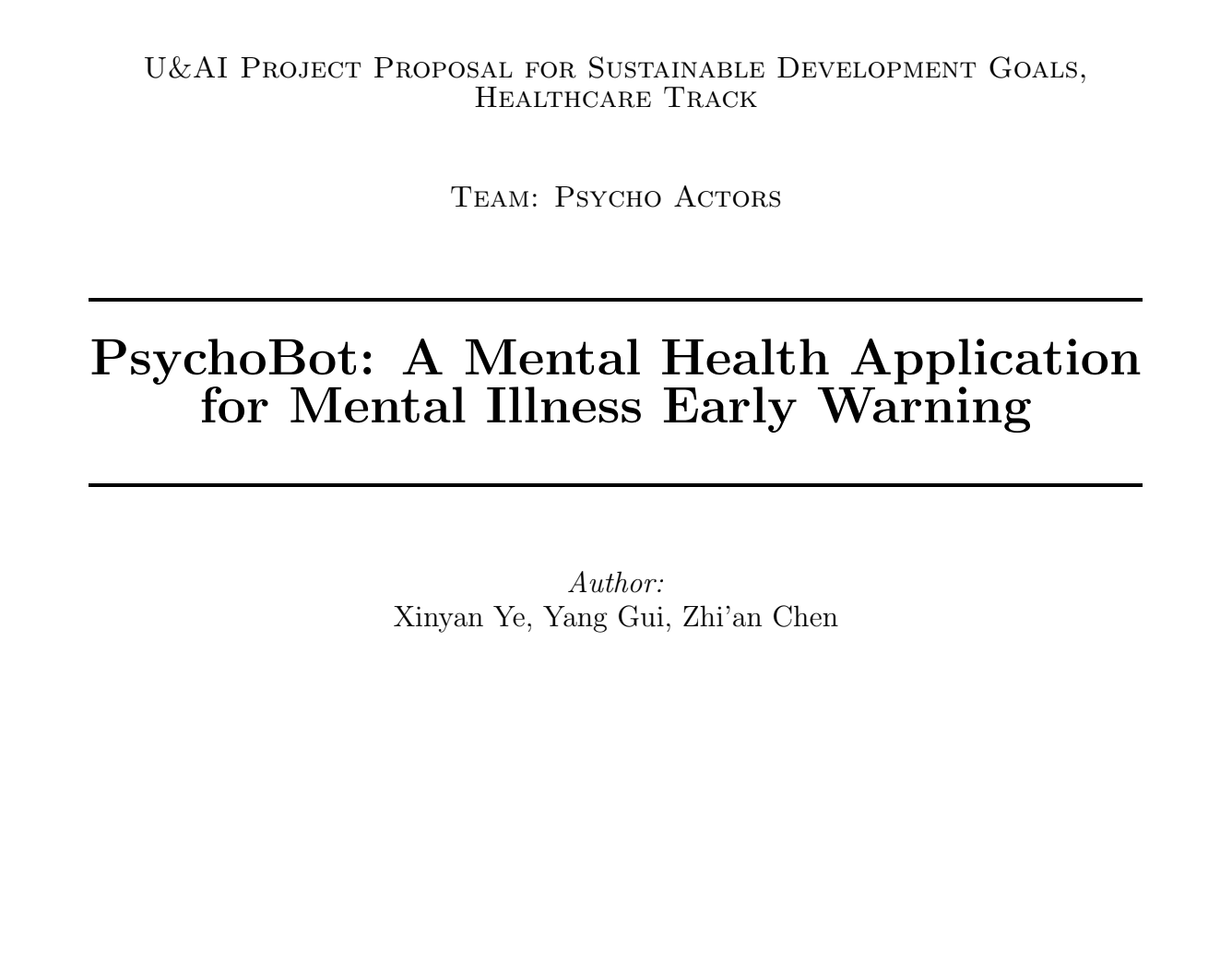
2022 summer, I led a team in designing a chatbot for detecting mental illness in the project proposal competition on AI for Sustainable Development Goals, co-orgnised by the Institute for AI International Governance of Tsinghua University and the United Nations Development Programme. Our mental healthcare application received universal praise and achieved a top 10 position out of 200+ teams in the competition.
Inspiration for the project comes from my research internship with Professor Abbas Edalat's Algorithmic Human Development Group, where I learned about the challenges of implementing AI in clinical practice, including user resistance to change and the variation in effectiveness among individuals. Our chatbot, which simulates a virtual psychological counselor featured with emotion recognition and compassionate language generation capabilities, aims to identify and address mental health issues early on and provide customized guidance for improving mental health literacy. In my career, I hope to raise awareness among healthcare professionals about the potential of AI to improve outcomes in healthcare settings and develop AI systems that can adapt to the diverse needs of patients.
Winner of 2021 Biomedical Data Science Challenge @ Imperial College Data Science Institute
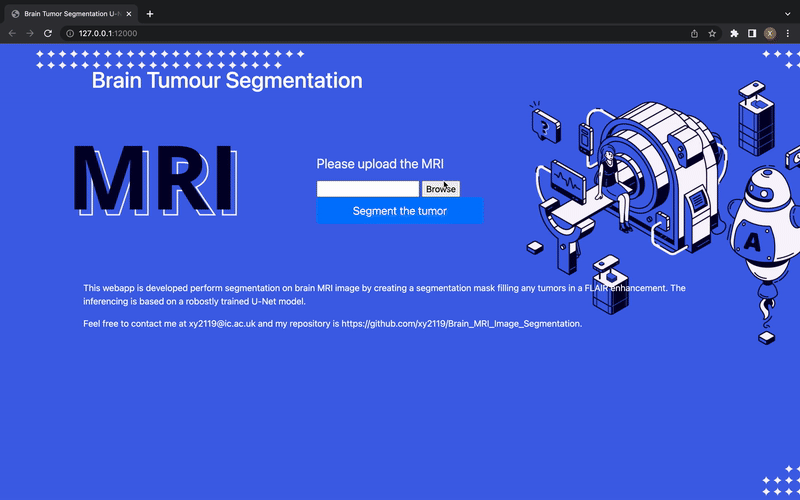
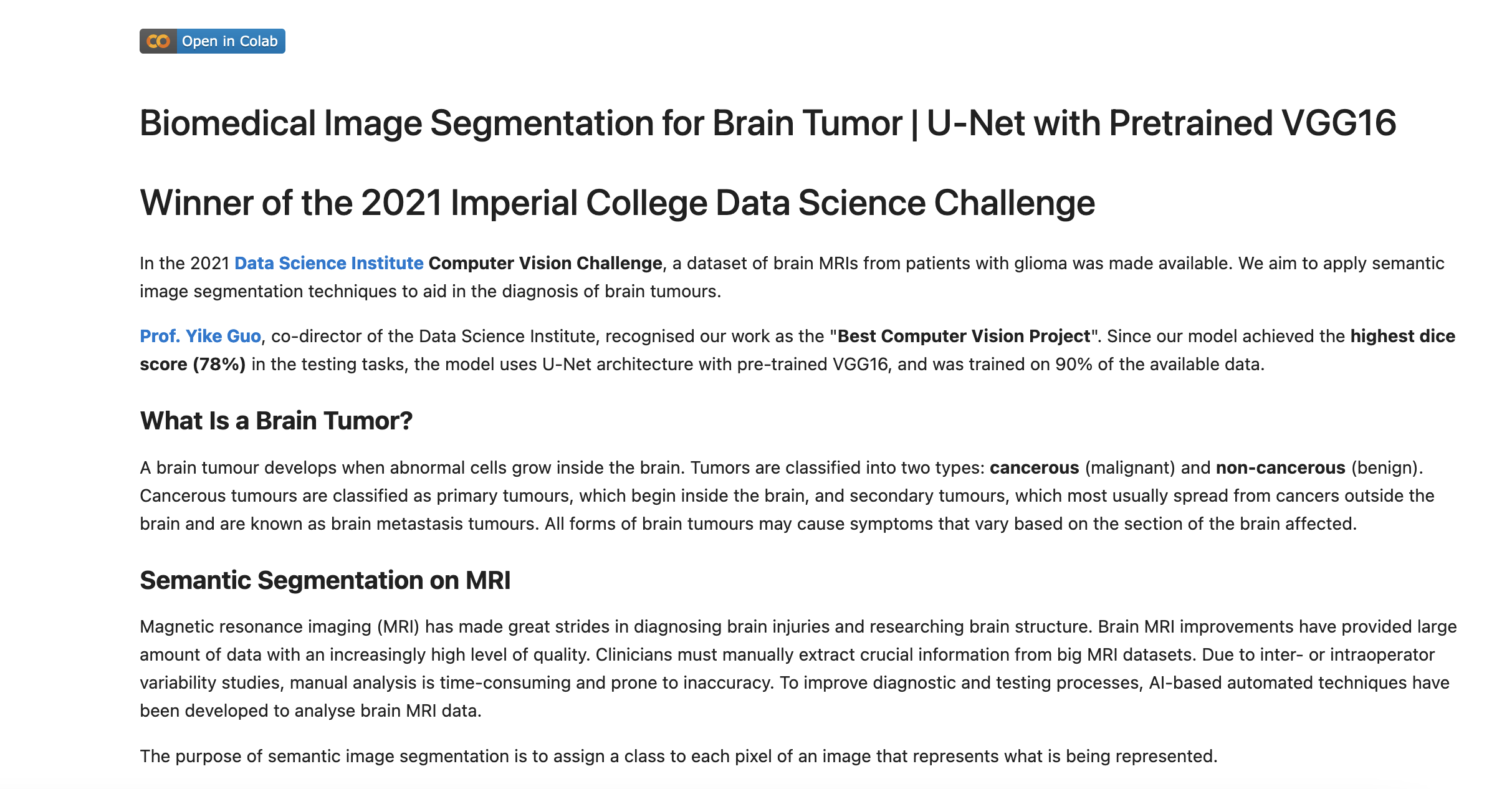
I developed an API for tumor segmentation based on an image segmentation model for the 2021 Data Science Institute Biomedical Data Science Challenge. The model performance was recognized by the Institute's co-director and I further turned it into a website for healthcare professionals to test with real cases. It means a lot to me.
I became interested in medical diagnostics after my cousin received an incorrect diagnosis of a grade 2 glioma. Six months after his surgery, he was able to return to his normal life and PhD studies in the UK. Upon further investigation, it was discovered that his tumor was an atypical encapsulated glioma, which should have been classified as a grade 1 according to the prevailing classification criteria at the time of his diagnosis. This incident made me realize the impact that limited resources and changing criteria can have on the accuracy of medical diagnoses and sparked my interest in precise diagnoses. I was acutely aware that if his doctors had made a wrong diagnosis, a common problem in the realm of medicine globally, this story would not have had a happy ending.